Search and Browse documents and HTR-Results in the Digital Library MV
We present our results in the Digital Library Mecklenburg-Vorpommern. Here you will find the digital versions with their corresponding transcriptions.
If you have selected a document– as here for example the Spruchakte of 1586 – you will then see its first page in the centre of the display. The box above allows you to switch to the next, the previous or any other page of your choice (1.). You can rotate the image (3.) zoom in or out (5.), choose two-page mode (2.) and switch to full screen mode (4.).
On the left side you can select different view options („Ansicht“). Here you can, for example, display all images at once instead of just one page („Seitenvorschau“) or you can read the transcribed text right away („Volltext“).
If you want to navigate in the structure of the file, first open the structure tree of the file in the bottom left box using the small plus symbol. Then you can select any given date.
Are you looking for a certain name, a place or some other term? Simply enter it in the search box on the left („Suche in: Spruchakte 1586“). If the term occurs in the file, the full-text hits („Volltexttreffer“), meaning all places where your search term occurs in the text, are indicated.
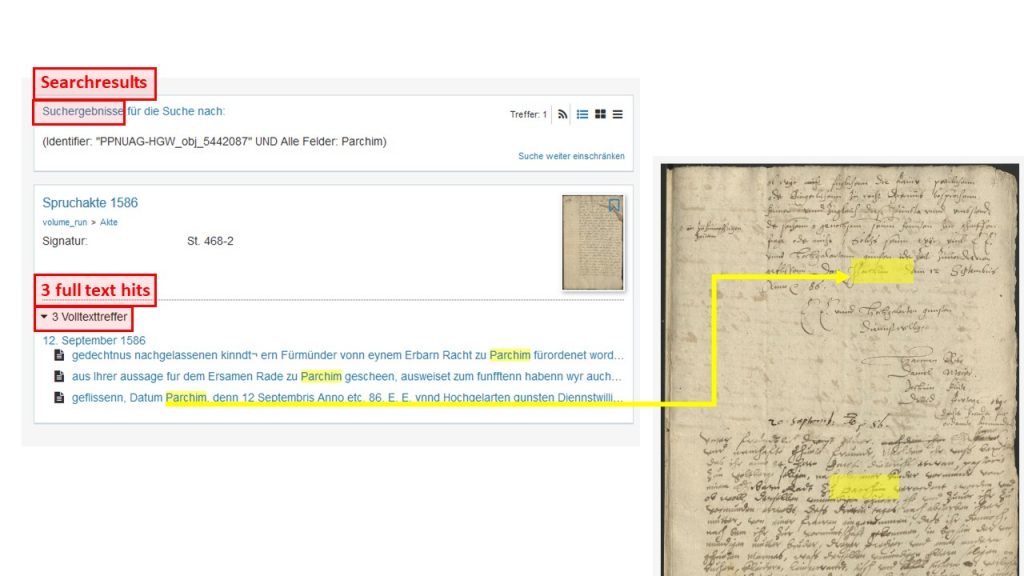
If you select one of the hits here, your search term will be marked yellow on the digital image. For now, highlighting the search result will only work on the digitized page, not yet in full text.
Tips & Tools
Display the found full text hits in a new tab (right mouse button). Navigating forwards and backwards in the Digital Library is still a bit tricky. This way you can be sure that you will always return to your previous selection.