Region Grouping
Since the version update 1.14.0 there is a new function to configure the layout analysis. It is about the arrangement of the text regions, called ‘Region grouping’. Now you can configure if they should be grouped around baselines or if all lines should be in one TR.
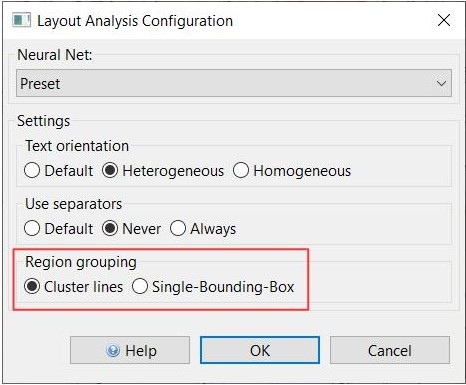
With the first mentioned setting it can happen that many small TRs appear at the edge of the image or in the middle of it, even if there is actually only one text block. This problem can be solved in a further step with the Remove small Textregions.
On the other hand, if only one text region is set, really all baselines are in this text region, even those that are otherwise marginal and even vertical BL. As long as the setting ‘Heterogeneous’ is selected for ‘Text orientation’, the layout analysis also recognizes the vertical lines in the same TR with the horizontal ones. It can be seen that the LA would normally recognize multiple TR. In fact, the reading order for the lines is still divided as if they were in their own text regions. The main paragraph is usually TR 1, so the RO starts there. The other baselines are placed at the back, even if they are at the side of the main text and could therefore be placed between them.
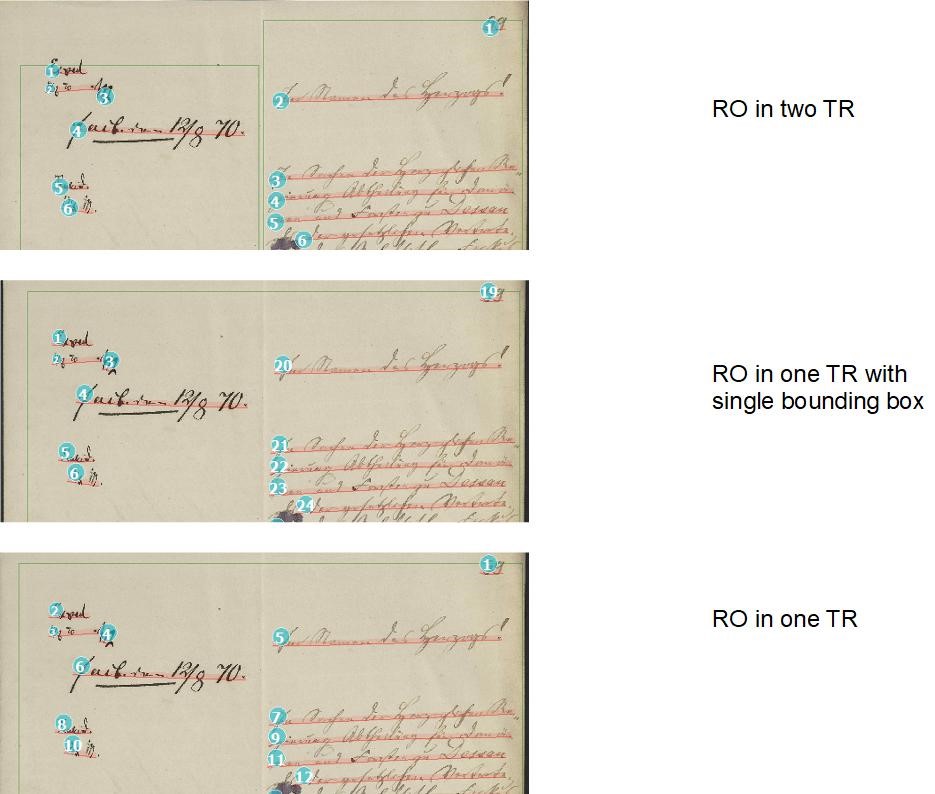
To decide which setting is better for you, you have to try it out. For pages that have only one text block, the second setting is of course advantageous, because all the small TR do not appear. It could also be that you have to choose different settings within one document.