Release 1.9.1
Medieval and early modern manuscripts are usually full of abbreviations in all possible variations. These can be contractions (omission in the word) and suspensions (omission at the end of the word) as well as a wide variety of special characters. So if we want to transcribe old manuscripts, we must first consider how we want to reproduce the abbreviations: Do we reproduce everything as it appears in the text, or do we resolve everything – or do we adapt to the capacities of the HTR?
Basically there are three different ways to deal with abbreviations in Transkribus:
– You can try to reproduce abbreviation characters as Unicode characters. Many of the abbreviation characters used in 15th and 16th century Latin and German manuscripts can be found in the Unicode block “Latin Extended-D”. For special characters written in medieval latin texts, check the Medieval Unicolde Font Initiative. It depends entirely on the goals of your own project whether and when this path makes sense – it is quite complex anyhow.
– If you don’t want to work with Unicode characters, you could also use the “basic letter” of the abbreviation from the regular alphabet – like a literal transcription. Such a “placeholder” can then be provided with a textual tag that marks the word as an abbreviation (“abbrev”). How the tagged abbreviation is to be resolved can then be entered for each tag as “expansion”.
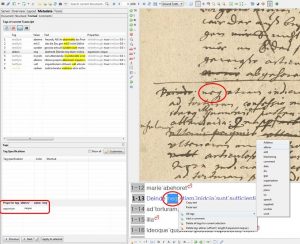
Thus the resolution of the abbreviation becomes part of the metadata. This approach offers the most possibilities for further use of the material. But it is also very laborious, because each and every abbreviation has to be tagged.
– Or you just dissolve the abbreviations. If you want to make large quantities of full text searchable, as we do, it makes sense to resolve the abbreviations consistently because it makes the search easier: Who is looking for “pfessores” instead of “professores”? We have made the experience that the HTR can handle abbreviations quite well; both the classic Latin and German abbreviations, as well as currency symbols or other special characters. This is why we resolve most abbreviations during transcription and use them as part of Ground Truth in HTR training.
The models we train have learned some abbreviations very well. The abbreviations frequently used in the manuscripts, such as the suffix “-en”, can be resolved by an HTR model – if it has been taught consistently.
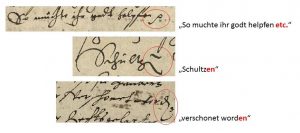
But more complex abbreviations, especially the contractions, do cause difficulties for the HTR. In our project we have therefore decided to reproduce such abbreviations only in literal form.
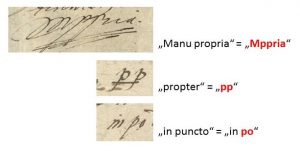
In our Collection of Abbreviations we present the many different abbreviations that we find in our material from the 16th to 18th century. We also show how we (and later the HTR models) resolve them. This Collection will be updated by us from time to time – work in progress!